Hypertune assistant: Simplify hyperparameter search processes
Boost model performance with hypertune assistant's intuitive tuning features, including customizable parameters and dataset inputs for optimized results.
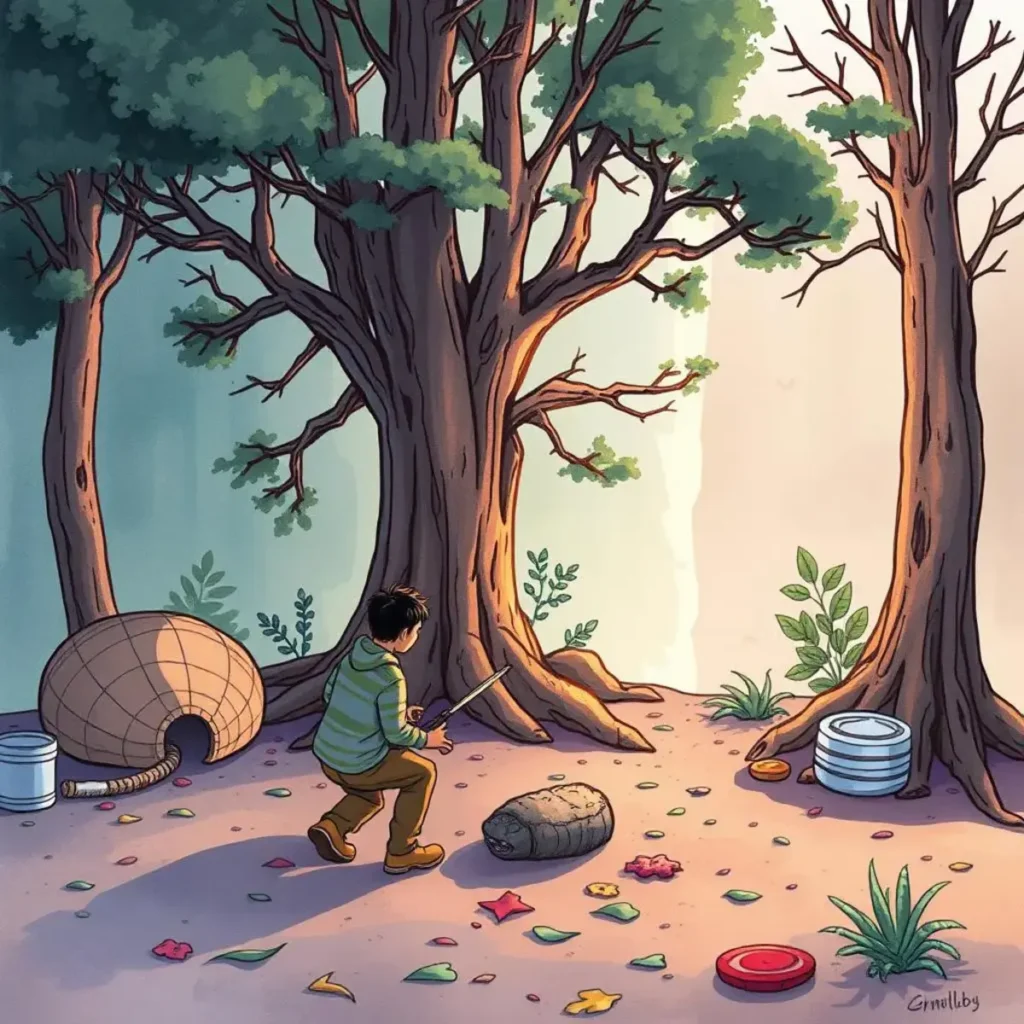
Fill out one or more form fields
Similar apps
Optimizing hyperparameter searches for enhanced model performance
Streamline your model retraining process with ease
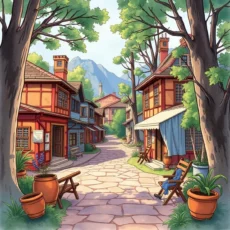
Robust input fields for precise data entry
Easily enter crucial information such as model identifiers, dataset paths, and hyperparameters to ensure that your model training is based on accurate and relevant data. This feature improves the quality of AI outputs by encompassing all necessary key details seamlessly.
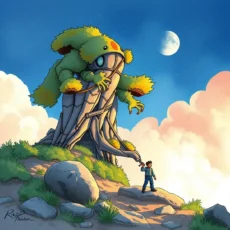
Customizable iteration and learning rate options
Input and adjust your desired number of tuning iterations along with tailored learning rate ranges to fine-tune your model effectively. This flexibility allows data scientists to explore various scenarios while optimizing performance metrics efficiently.
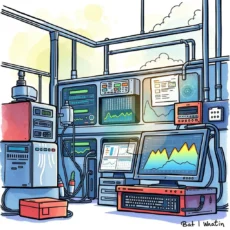
Flexible batch size configuration
Enter multiple batch size options, such as 16, 32, or 64, allowing users to experiment with differing configurations to achieve optimal model performance. This feature accommodates a wide array of processing environments for improved experimentation.
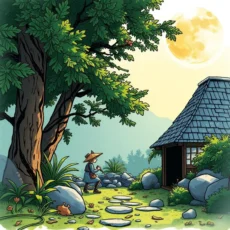
Advanced regularization parameter integration
Specify L1 or L2 regularization values directly in the app to help mitigate overfitting during model training. Implementing these parameters ensures a robust result while enhancing overall accuracy for your model predictions.
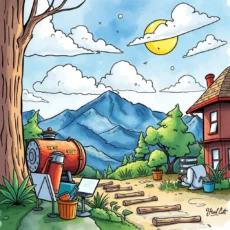
Evaluative metric setup for enhanced performance tracking
Select from various evaluation metrics like accuracy or F1 score to measure and analyze your trained models effectively. This capability enables ongoing assessments throughout the tuning process, guiding improvements and refining outcomes systematically.
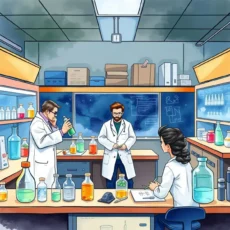
Random seed allocation for reproducibility
Enter a random seed value to guarantee consistent results across multiple training runs. This critical feature promotes reproducibility in experiments, ensuring that findings can be verified and built upon by both individual users and teams alike.
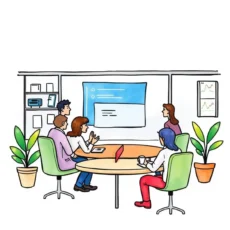
Incorporate additional hyperparameters seamlessly
Flexibly include any supplementary hyperparameters such as dropout rates in your tuning process. This functionality empowers data scientists and machine learning engineers to tailor their models further for improved adaptability and performance outcomes.
Additional information
Best for: Machine Learning Engineer, Data Scientist, AI Research Scientist, Model Optimization Specialist, Hyperparameter Tuning Specialist