Adaptive tuner: Dynamically adjust hyperparameters based on feedback
The adaptive tuner simplifies model retraining, enabling quick and effective hyperparameter tuning using your dataset for better machine learning outcomes.
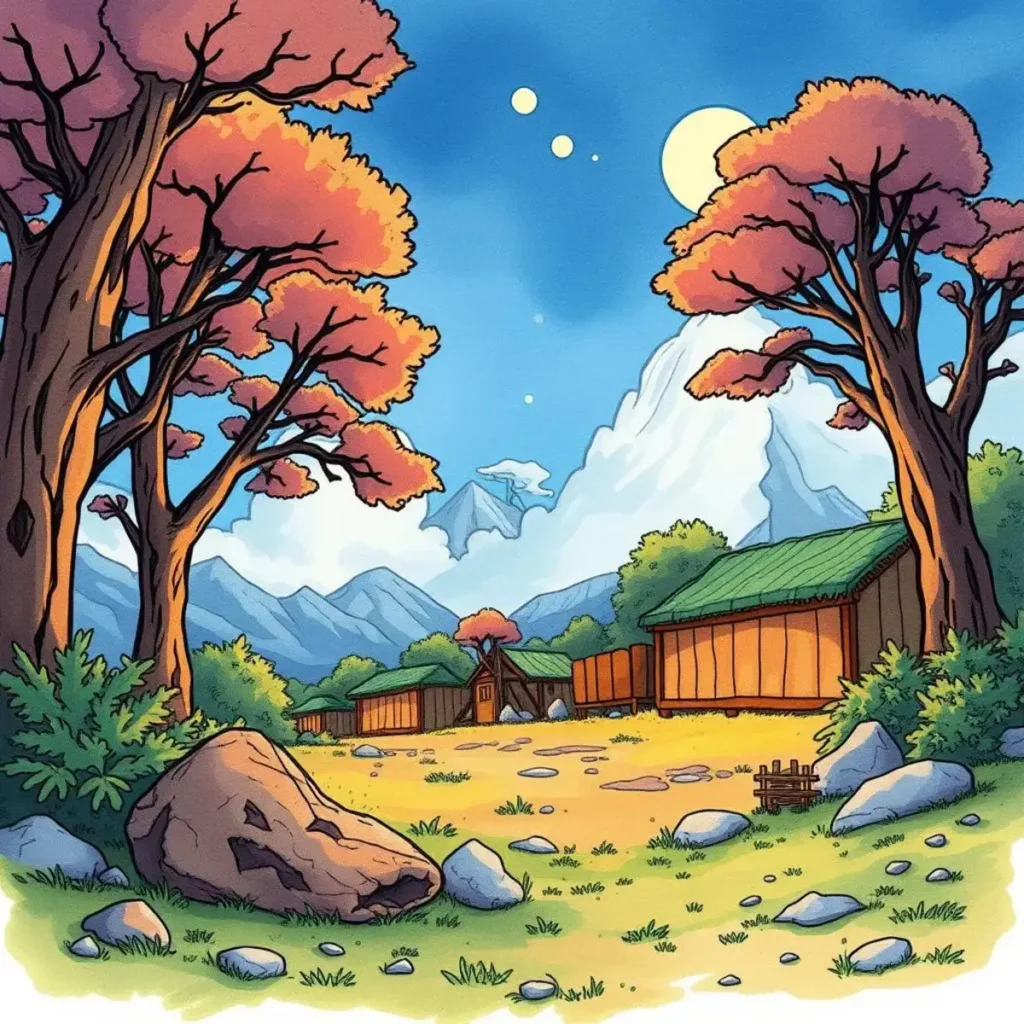
Fill out one or more form fields
Maximize model performance with unique adaptive tuner features
Elevate your analytics and data science tasks today
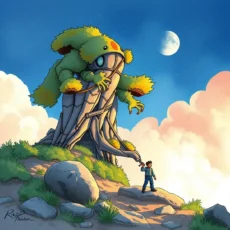
Enter comprehensive model information
Inputting essential model data, including name, dataset, iterations, and learning rate, allows for comprehensive hyperparameter tuning. Each field is designed to capture critical details that directly influence model performance and accuracy. Enhance your results by ensuring all key parameters are provided for optimal output quality.
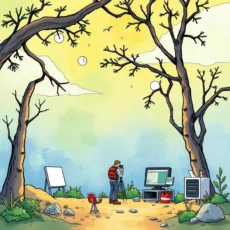
Optimize learning process with key parameters
Seamlessly adjust variables such as batch size, regularization parameter, and evaluation metrics. Each tweak plays a vital role in refining your machine learning model's performance. The ability to customize these parameters ensures tailored results that meet the unique needs of your datasets.
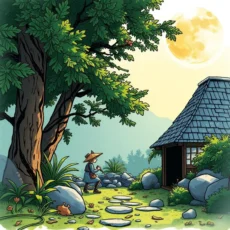
Choose effective tuning methods
Select from proven tuning methods like grid search or random search to find the best hyperparameter settings efficiently. This feature simplifies the exploration process, allowing data scientists to quickly identify optimal configurations that enhance model accuracy without unnecessary complexity.
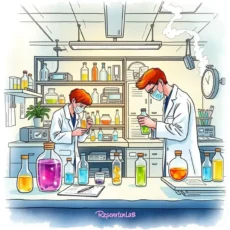
Enhance experiment reliability with validation tools
Utilize capabilities for entering validation dataset names and random seed values to maintain experiment integrity. These features are critical for reproducibility and reliability in research, enabling data scientists to ensure consistent results across different experiments.
Similar apps
Additional information
Best for: Machine Learning Engineer, Data Scientist, AI Engineer, Model Optimization Specialist, Hyperparameter Tuning Specialist